Vu Anh Le
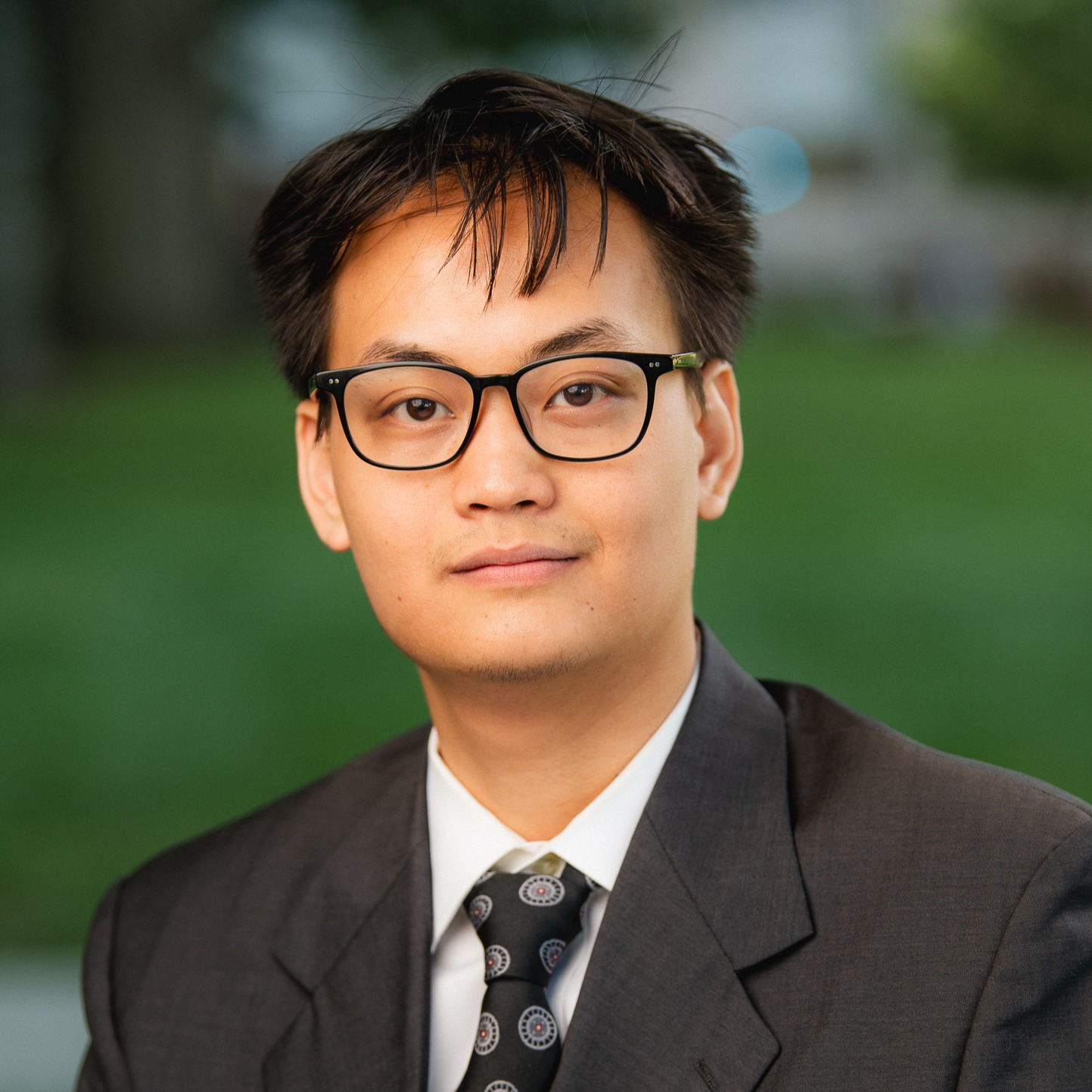
MIT Department: Nuclear Science and Engineering
Faculty Mentor: Haruko Murakami Wainwright
Undergraduate Institution: Beloit College
Hometown: Hanoi, Vietnam
Website: LinkedIn
Biography
Vu-Anh Le is a rising senior at Beloit College, working towards a B.Sc. in Mathematics. Driven by a profound interest in computational sustainability, he aspires to undertake graduate studies in computational science and engineering, focusing on designing, implementing, and popularizing models to solve environmental and alternative infrastructure challenges. As an MSRP intern, he works under the mentorship of Prof. Haruko Murakami Wainwright in the Department of Nuclear Science and Engineering, developing data-driven models for long-term monitoring strategies of radioactively contaminated Superfund sites. He is also a long-term collaborator with Vietnam’s Ministry of Natural Resources and Environment, applying statistical modeling to reveal land deformation trends in Vietnam and the impact of hydroelectric power construction on these trends and local livelihoods. These experiences have influenced him to pursue a career as a computational scientist, public interest attorney, and government consultant—accelerating the progress toward the United Nations’ SDGs. In his leisure time, he participates in cross country, long-distance track and field, and reading human history.
Abstract
Assessing the Site Closure Time Frame for Soil and Groundwater
Contaminated Sites
Vũ Anh Lê1, 2, 3, Haruko Murakami Wainwright2, 3
1Department of Mathematics and Computer Science, Beloit College
2Department of Nuclear Science and Engineering, Massachusetts Institute of Technology
3Department of Civil and Environmental Engineering, Massachusetts Institute of Technology
Monitored Natural Attenuation (MNA) is increasingly recognized for its cost-effective and minimally invasive approach to managing soil and groundwater contamination. Utilizing the PyLEnM machine learning framework, we developed a data-driven model to estimate the time for contaminants like Sr-90 and I-129 to reach regulatory safety standards. The study integrates linear regression, random forest regression, and an ensemble deep neural network model to analyze trends in contaminant concentrations, accounting for the site’s complex hydrostratigraphic units and geochemical conditions. Preliminary results indicate a significant downward trend in contaminant levels, with the maximum time to reach safety thresholds being 320 years for Sr-90 and 258 years for I-129 amongst decreasing wells. Our findings highlight the importance of specific hydrological factors, such as total depth and screen zone, in enhancing the predictability and effectiveness of MNA strategies, ensuring long-term groundwater protection. The study scope covers the Savannah River Site (SRS), a Department of Energy-owned Superfund site contaminated with radionuclides that plans to apply MNA.