Nathan Zekarias
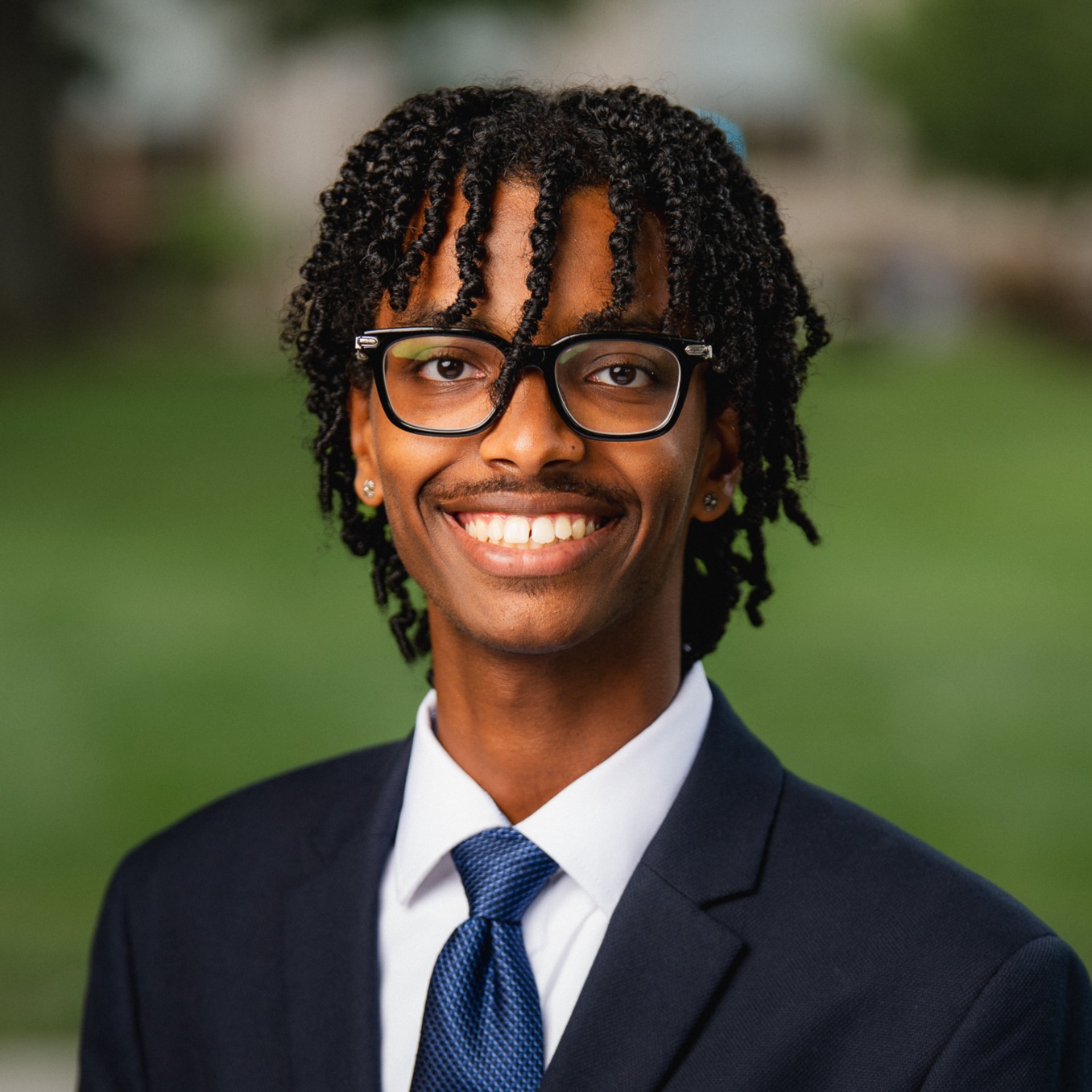
MIT Department: Electrical Engineering & Computer Science
Faculty Mentor: Prof. Marzyeh Ghassemi
Research Supervisor: Walter Gerych, Cassandra Parent
Undergraduate Institution: University of Maryland, Baltimore County
Hometown: Silver Spring, Maryland
Website: LinkedIn
Biography
Nathan Zekarias is a rising senior at the University of Maryland, Baltimore County studying Biology and Computer Science. Since he was young, he asked endless questions to those around him and was encouraged to experiment to find the answers. This led him to join the Meyerhoff Scholars program, where his peers also encouraged this practice. These experiences, along with studying abroad, led him to pursue a wide variety of research experiences. These experiences include studying bone-integrated prosthetics at UMD and studying invasive and native species relationships in Puerto Rico. This summer, he is training machine learning models to predict the vulnerability of people in the United States. With this range of experiences, Nathan is able to holistically look at issues and use multiple perspectives to answer interdisciplinary research questions. It is with this wide range of experiences that Nathan was able to decide to pursue a PhD in Computer Science.
Abstract
Predicting Social Determinants of Health Using Machine Learning on
Environmental Data
Nathan Zekarias1, Cassandra Parent2, Dr. Walter Gerych3, Professor Marzyeh Ghassemi3
1Biological Sciences/Computer Science, University of Maryland – Baltimore County
2IMES, Massachusetts Institute of Technology
3CSAIL, Massachusetts Institute of Technology
Social determinants of health (SDOH) are nonmedical factors that affect an individual’s health.
The HealthyML group at MIT is studying the relationship between SDOH and environmental
health in an effort to foster healthier communities. The group uses environmental data such as
species diversity and land cover data to predict SDOH at a census tract level. The aim is to show that improved environmental health has a positive effect on SDOH, which will enable human health predictions as environmental health changes. The Social Vulnerability Index (SVI) is an index developed by the CDC to quantify SDOH factors that have adverse effects on
communities. This project trained machine learning models like XGBoost, Random Forest, and
Logistic Regression on environmental data sets and observed if they could predict the SVI within a threshold. Data sources for the feature set were derived from the CDC, iNaturalist, EPA, and MRLC, which were separated at the census tract level. In Maryland, SVI was successfully predicted from the environmental data (AUC = 0.85 from XGBoost), with land cover like hay pastures being a strong indicator for low vulnerability, indicated by a lower SVI. These methods can be propagated country-wide to define how environmental health impacts human health.