Montgomery Bohde
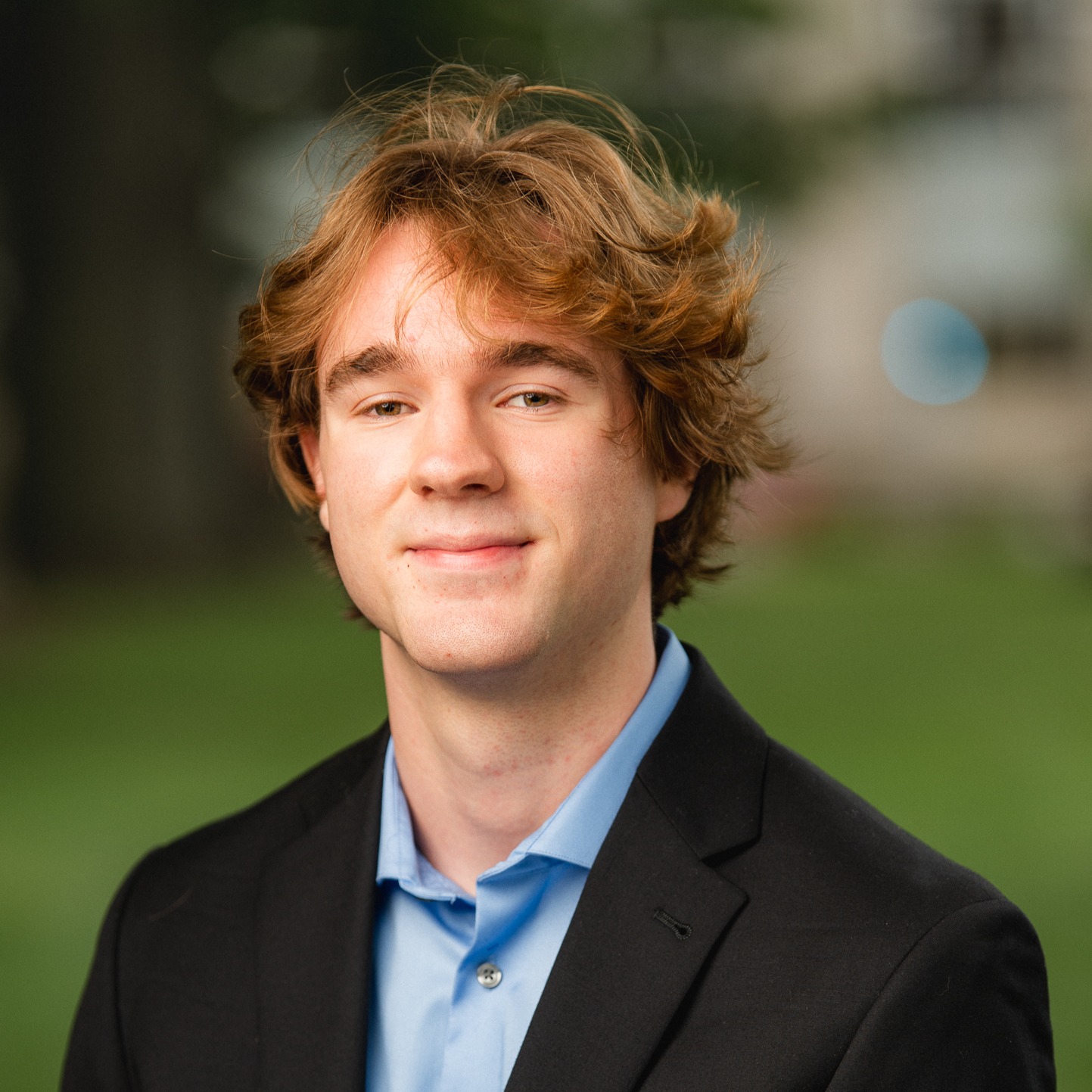
MIT Department: Chemical Engineering
Faculty Mentor: Prof. Connor Coley
Research Supervisor: Runzhong Wang
Undergraduate Institution: Texas A&M University
Hometown: Plano, Texas
Website: LinkedIn
Biography
Montgomery Bohde is a rising Junior at Texas A&M University, double majoring in Computer Science and Applied Math. Growing up, Montgomery was fascinated by biology, and his research interests now lie at the intersection of machine learning, biology, and chemistry. Montgomery started doing research during his freshman year, where he worked on developing machine learning models for molecule-material interactions. His main research interests revolve around building powerful and efficient deep learning models. He is especially interested in building deep neural networks for modeling molecules and proteins. Outside of research, Montgomery is involved in many student organizations on campus, including serving as the Technical Director of Texas A&M Computing Society. After graduating from Texas A&M, he plans to pursue a PhD in Computer Science and a career as a research scientist in industry.
Abstract
A Deep Generative Model for Tandem Mass-Spectra Structure Elucidation
Montgomery Bohde1, Runzhong Wang2, Connor W. Coley2,3
1Department of Computer Science and Engineering, Texas A&M University
2Department of Chemical Engineering, Massachusetts Institute of Technology
3Department of Electrical Engineering and Computer Science, Massachusetts Institute of
Technology
Metabolomics studies have identified small molecules that mediate cell signaling, competition and disease pathology, in part due to large-scale community efforts to measure tandem mass spectra for thousands of metabolite standards. Nevertheless, the majority of spectra observed in clinical samples cannot be unambiguously matched to known structures. In recent years, several deep learning approaches have been used for mass spectra structure elucidation, however they rely on database search, which is both inefficient and incapable of identifying molecules which are not already in known structure databases. In this work, we introduce a deep generative model for untargeted metabolomics, Deep Diffusive Metabolite Generator (DDMG), to directly generate molecular structures that match a given mass spectra. Unlike existing methods, DDMG does not rely on structural database search, allowing it to generalize to the search space of all potential metabolites. To generalize well when data is limited, we computationally generate approximate mass spectra readings for a large structural database. We then use this noisy data for pre-training DDMG before finetuning the model on experimental data. We experimentally validate the robustness of this new structure elucidation pipeline and show that DDMG can correctly generate structures unseen in the training datasets.