David Flores
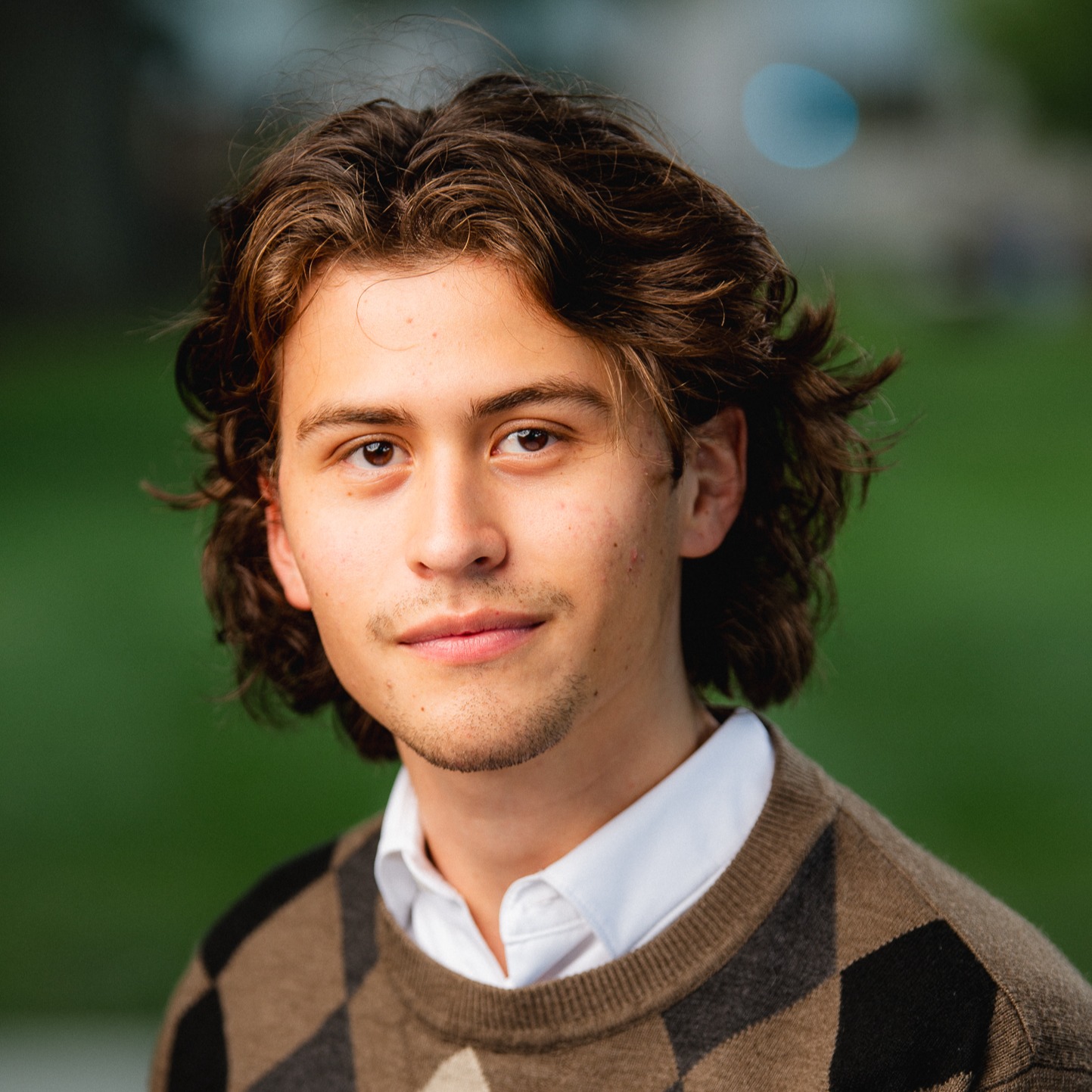
MIT Department: Aeronautics and Astronautics
Faculty Mentor: Prof. Nicholas Roy
Research Supervisor: Erick Fuentes, Ethan Fahnestock
Undergraduate Institution: University of New Mexico
Hometown: Las Cruces, New Mexico
Website: LinkedIn
Biography
David Flores is a rising junior at the University of New Mexico, majoring in Electrical Engineering with a concentration in control systems engineering. Throughout his college career, David has been actively engaged in robotics research and community projects beginning in the summer of 2023 in the collaborative robotics lab at Purdue University, where he made a modular sensing platform for detecting methane production in farm cattle. At UNM, he was the president of the robotics club for over a year, focusing on both competition robotics and community outreach efforts. Most recently, David has contributed to the robust robotics group at MIT, exploring methods for path planning under position uncertainty. Apart from robotics, David conducts research in the Human-centered Autonomy and Controls Lab at the University of New Mexico and serves as a peer tutor for math and physics. With a demonstrated track record of leadership and a passion for academic growth, David is committed to pursuing a PhD in engineering and working towards advancing control algorithms in legged robots, while also exploring methods for optimizing for a more natural gait.
Abstract
Comparison of Motion Planning Algorithms That Utilize Probabilistic Decision Making Weighted on Landmark Density in an Environment
David J. Flores1, Nicholas Roy2, Erick Fuentes2
1Department of Electrical and Computer Engineering, University of New Mexico
2Department of Aerospace and Astronautics, Massachusetts Institute of Technology
In recent years, first responders have been utilizing robots more frequently for search and rescue missions when environments are inaccessible, obstructed, or dangerous. While this is a great first step in mitigating risk, navigating and path planning through unpredictable situations becomes difficult when wireless signals transmitted to the robot are attenuated or there are physical obstructions to its non-optical sensors. Thus, for when knowledge of the robots position and orientation is uncertain – such as GPS denied environments – we have opted to shift towards using external landmarks in the environment for helping the robot localize itself. As the nature of any environment is somewhat stochastic, we optimize for end-state certainty through the use of the known probabilistic landmarks. A current method of handling these noisy factors in planning has been to include a prediction of future uncertainty and state estimates using extended Kalman filtering which has proven to lead to better motion performance. My lab has previously worked on reducing the computational cost of these probabilistic position estimates by using a factored form of the covariance matrix and dubbed the method the “The Belief Roadmap”(BRM). I have extended upon this research by designing three separate planning algorithms that incorporate some knowledge of the environment to make informed, yet weighted and randomized, choices for future states. We would like to first see how pseudorandom exploration computationally compares to BRM as well as determine the planners’ efficacies at increasing goal state certainty.