Chelsea Joe
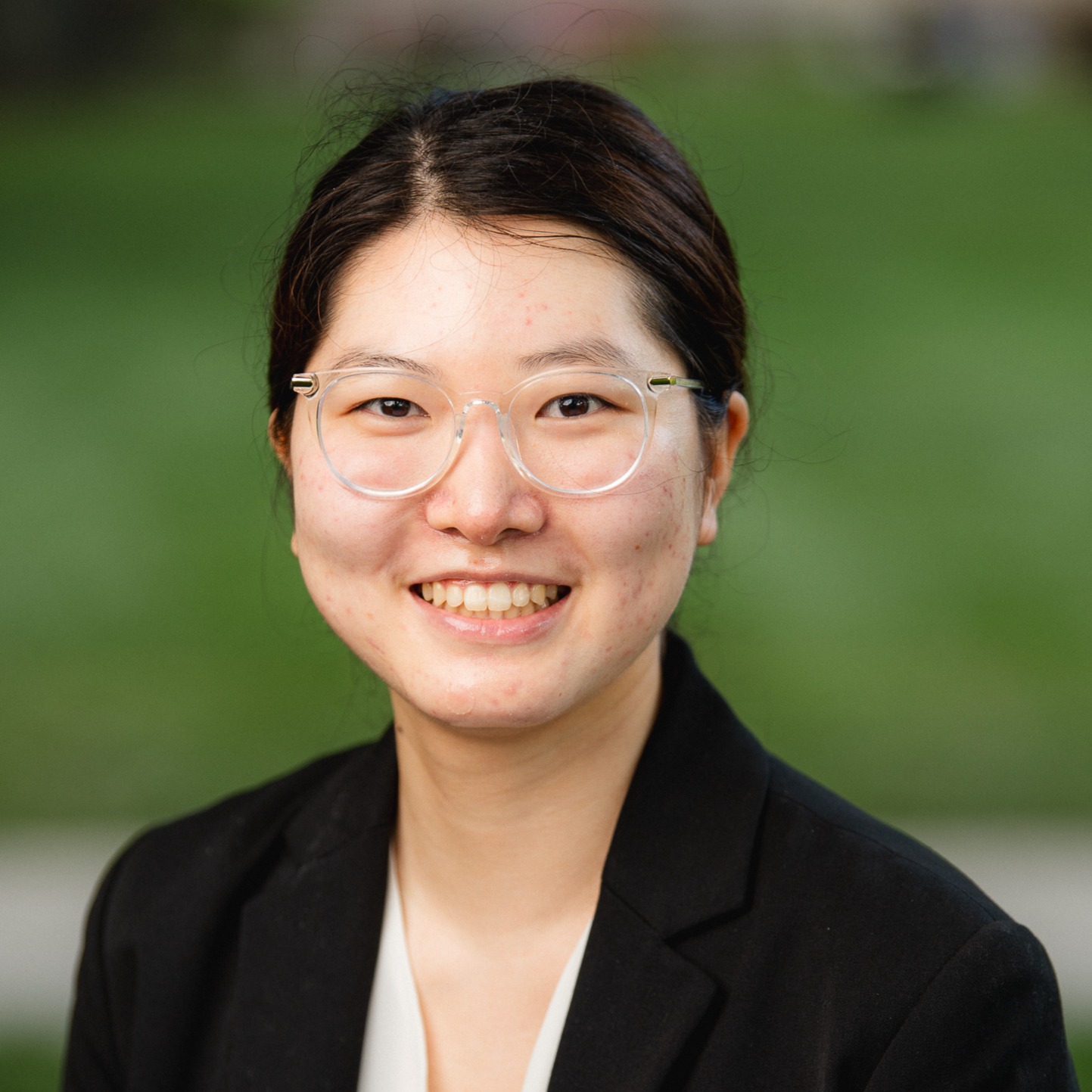
MIT Department: Media Arts and Sciences
Faculty Mentor: Prof. Cynthia Breazeal
Research Supervisors: Safinah Ali, Hae Won Park
Undergraduate Institution: Dartmouth College
Hometown: Las Vegas, Nevada
Website: LinkedIn, Intern’s Website
Biography
Chelsea Joe is a rising senior at Dartmouth College, double majoring in Computer Science and Neuroscience. Her passion lies at the intersection of Artificial Intelligence, Wearables, and Robotics—inhuman tools that can be leveraged to support meaningful human connections. Chelsea aims to apply her interests in Natural Language Processing and Human-Computer Interaction to develop technologies in the fields of human cognition and AI to enhance the human experience. She received the 2024 Activision Blizzard King Inclusion in Gaming Scholarship and has participated in the Meta ABCS Program, the CodePath Development Program, and the Apple Pathways Alliance Program. As an MSRP intern in Personal Robots at the MIT Media Lab, her project focuses on how Large Language Models can analyze parent-child interactions to generate effective parenting strategies. During the summer, she aims to gain more experience in AI and HCI research and make meaningful connections.
Abstract
Learning to Parent: Personalized Strategies for Parent-Child Interaction
Chelsea Joe1, Hae Won Park2 and Cynthia Breazeal2
1Department of Computer Science, Dartmouth College
2Department of Media Arts and Sciences, Massachusetts Institute of Technology
Traditional Reinforcement Learning (RL) methods in robotics are resource-intensive and lack flexibility across various scenarios. This project explores a new approach utilizing Large Language Models (LLMs) to analyze parent-child interaction data and generate high-level insights for personalized parenting strategies. The primary goal is to develop a framework that collects episodes of parent-child interactions, group similar episodes, and extract high-level insights from them. The approach focuses on real-time insight extraction – when similar episodes are encountered, relevant insights are retrieved and updated, enabling generalization to various scenarios even with limited data and context. To achieve this, an experiential learning framework was developed that dynamically adapts and generalizes insights from limited data to different scenarios. Additionally, this framework will recognize meaningful interaction episodes in real-time, allowing for immediate analysis and response. The insights generated from this data is stored in a structured database and utilized for Retrieval-Augmented Generation to create personalized parenting strategies. The resulting model learns the strategies of parental dialogic and negotiation behaviors, trains on the collected insights, and ultimately generates personalized parenting strategies tailored to each parent’s unique style.