Aden McKinney
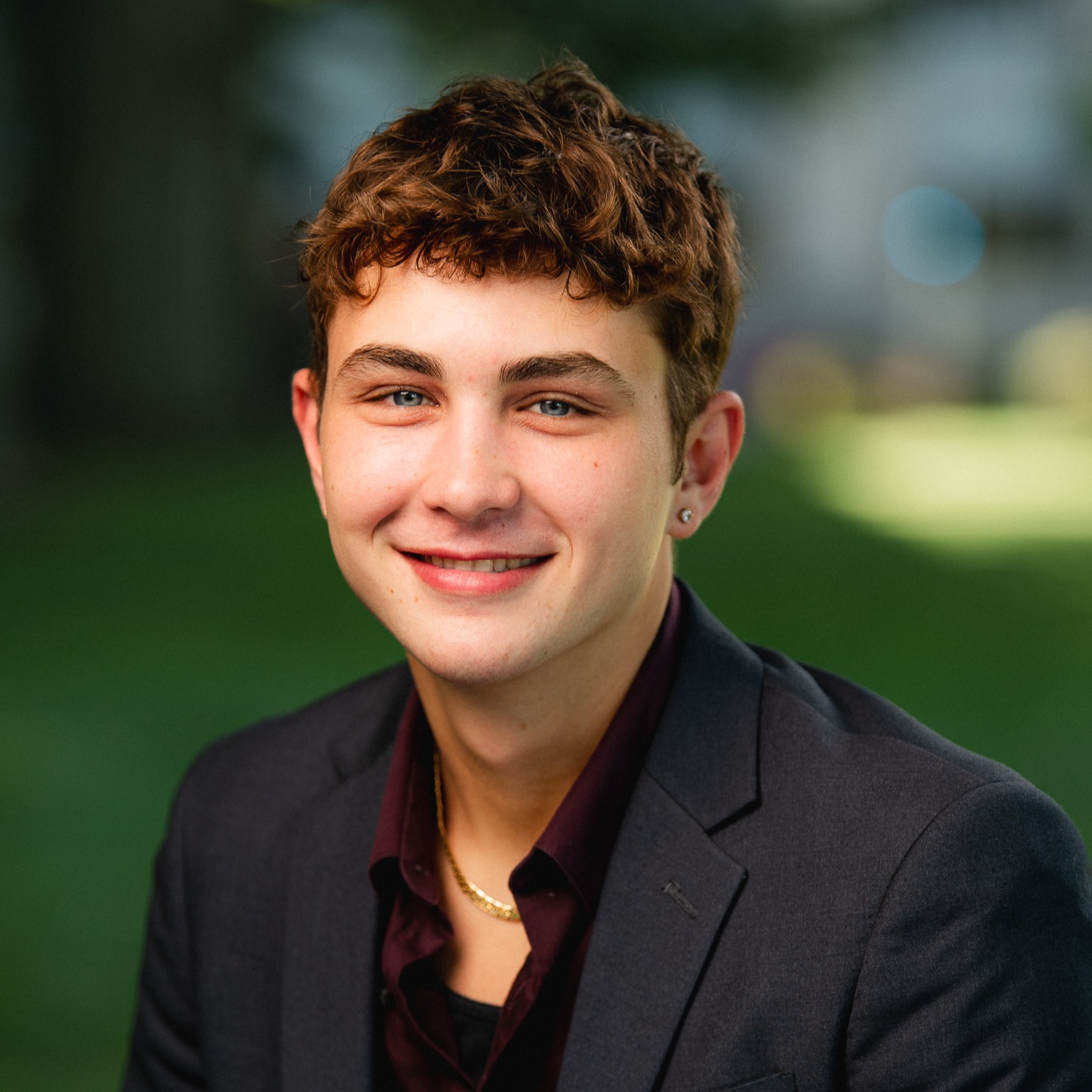
MIT Department: Electrical Engineering and Computer Science
Faculty Mentor: Prof. Navid Azizan
Research Supervisor: Sunbochen Tang
Undergraduate Institution: University of Central Florida
Hometown: Sarasota, Florida
Website: LinkedIn
Biography
Aden McKinney is a rising senior (‘25) at the University of Central Florida studying Computer Engineering with a background and interest in autonomous robotics. At UCF, he is actively involved in the IEEE student chapter as a previous president, and conducts reinforcement learning research under Dr. Chinwendu Enyioha. From this work, Aden had the opportunity to work as a robotics systems engineering intern at the NASA Jet Propulsion Laboratory, where he was first exposed to autonomous robotics research. Aden has applied this leadership, research, and engineering experience to implement and analyze learned controllers for autonomous drone flight under Dr. Navid Azizan while in MSRP. Looking ahead, Aden aims to join an autonomy-based PhD program and return to the space-bound robotics industry as a research scientist. Beyond his technical pursuits, Aden is passionate about promoting equitable STEM education and enjoys hiking, traveling, and watching Miami Heat basketball.
Abstract
An Empirical Analysis of Control-Oriented Meta-Learning Using Automated Mirror Descent
Aden McKinney1, Sunbochen Tang2, and Navid Azizan3
1 Department of Electrical and Computer Engineering, University of Central Florida
2 Department of Aeronautics and Astronautics, Massachusetts Institute of Technology
3 Department of Mechanical Engineering, Massachusetts Institute of Technology
The ability to adapt to unforeseen environmental dynamics is vital for the precise autonomous flight of drones. The control-oriented meta-learning (COML) algorithm, and its subsequent predecessor’s, achieve this adaptability through two steps: first, the offline learning of disturbance representations, and second, online adaptive control based on these learned models. This project analyzes the empirical performance of using Automated Mirror Descent for the optimization of the COML algorithm, with an emphasis on studying the observed generalizability to different kinds of environmental disturbance. This analysis tests the algorithm’s ability to track a reference trajectory in 3D space on a hexarotor drone platform. Performance is quantified and compared via a model’s cumulative tracking error when following a trajectory that intersects with a variety of disturbances. We envision the algorithm can further enable the autonomous flight of unmanned aerial vehicles (UAVs) due to the significantly improved performance observed when comparing COML to other common flight controllers of today.